Goal and Purpose
SUPRA is an international ZIM innovation network and is made up of a community of industrial and research partners from the field of production technology.
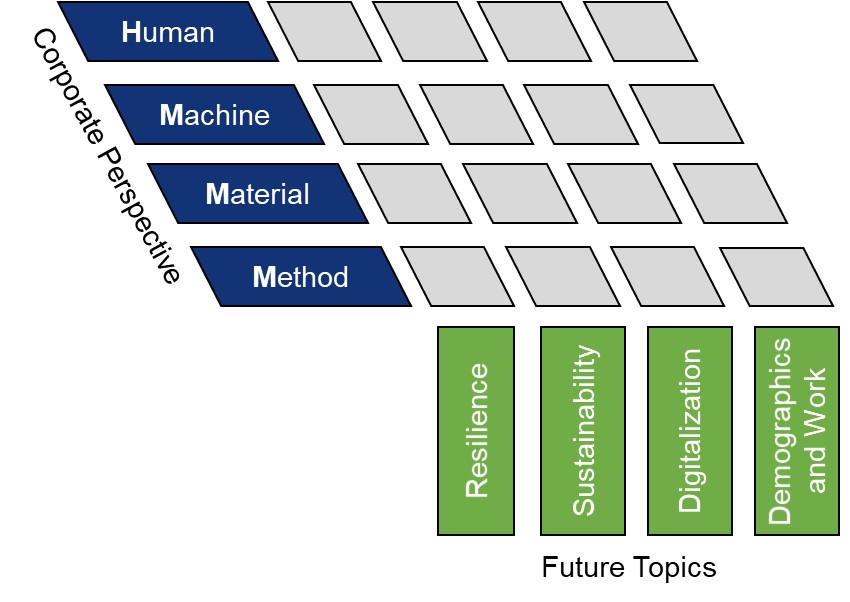
The goal of the network is the development of strategies to increase the resilience and sustainability of production processes through automation and the further development of production systems and components. Current challenges such as climate change, a shortage of skilled workers and increasing pressure to innovate and compete are particularly challenging for many companies and institutions. The central action matrix for the content-related orientation of the network is derived from this. This consists of an internal company perspective which is compared with identified future topics.
Through interdisciplinary and international cooperation, competencies, ideas and know-how are to be identified, developed and bundled. The activities are aligned with the "Agenda 2030 for sustainable development" of the United Nations. Taking up this idea of sustainability, developing it further and applying it specifically in the area of small and medium-sized manufacturing companies (SMEs) is the vision of SUPRA.
Lines of Development
The joint research of the SUPRA network is divided into the following six main areas:
1. Agile Manufacturing Processes and Systems
The increasing individualization of complex mass-produced products and ever shorter product life cycles require production and automation technology to develop adaptable and flexible manufacturing processes, including the associated manufacturing systems and system components. Manufacturing and disassembly process chains must be adapted and further developed to meet these requirements. In particular, the supplementation of existing machine concepts by the use of robots as well as the development of alternative machine kinematics using industrial robots should meet this pressure to adapt with regard to different target criteria (sustainability, quality, ...).
2. Environmental Economics in Production Technology
Increasing social demands on corporate action with regard to ecological aspects require greater attention to be paid to process chains and products. Their often still unknown environmental-economic interrelationships as well as their internal and external impact on the company require supplementation by an environmental management system and its transparent reporting. Derived from this are opportunities for action that enable the development of sustainable business models as well as products.
3. Human-Machine-Robot Cooperation
For complex manufacturing tasks and interlocked process chains, the cooperation of machine and robot lends itself. This inevitably leads to a multitude of questions starting with the underlying scientific methods and models up to the practical realization. The allocation of the respective manufacturing tasks to one of the two machine systems as well as their parallel or sequential processing, the consideration of the respective achievable, position- and workspace-dependent accuracy, dynamics and costs are also decisive economic restrictions. Furthermore, mutual process influences as well as questions of practical implementation have to be clarified. It is also necessary to evaluate the role of humans in this ecosystem, to consider the potentials and needs of interaction and to map the new distribution of tasks transparently.
4. Intelligent Data and Models
For sustainable processes and systems, condition detection, assessment and control are essential. The foundation for this is a broad knowledge base. The goal is to bring together a wide variety of heterogeneous information. Additional added value can be derived from this through data compression. Thus, in addition to process-accompanying monitoring and models for risk, error and potential analysis, conclusions can also be drawn from a bidirectional coupling of real system and model.
5. Machine Learning
Machine learning methods are particularly suitable for the formalization of non-trivial relationships. Their description, data preprocessing and merging as well as the development of individual algorithms serve to solve application-specific problems. The right choice of methodology (supervised learning, unsupervised learning and reinforcement learning) as well as the data quality are of central importance for the meaningfulness of the results. Furthermore, the variety of data to be considered, such as sensor, image and machine data, shows complexity of the subject area.
6. Smart Components and Sensors
Beyond classic monolithic construction materials, there is great potential in the use of functional materials, most of which have extraordinary properties. Examples include shape memory alloys that remember their original shape even after severe plastic deformation, auxetic structures whose design achieves a negative transverse contraction coefficient, or electrorheological fluids that enable switchable optical transmission when an electrical voltage is applied. These materials are often referred to as smart materials and can be used for actuator and/or sensory functions when integrated into semi-finished products or components.